Tripling Efficiency with Astronomer: How McKenzie Intelligence Services Transformed Catastrophe Assessment to 24/7 Operations
How McKenzie Intelligence Services Used Astronomer to Eliminate Manual Processes, Improve Data Consistency, and Enhance Global Disaster Response
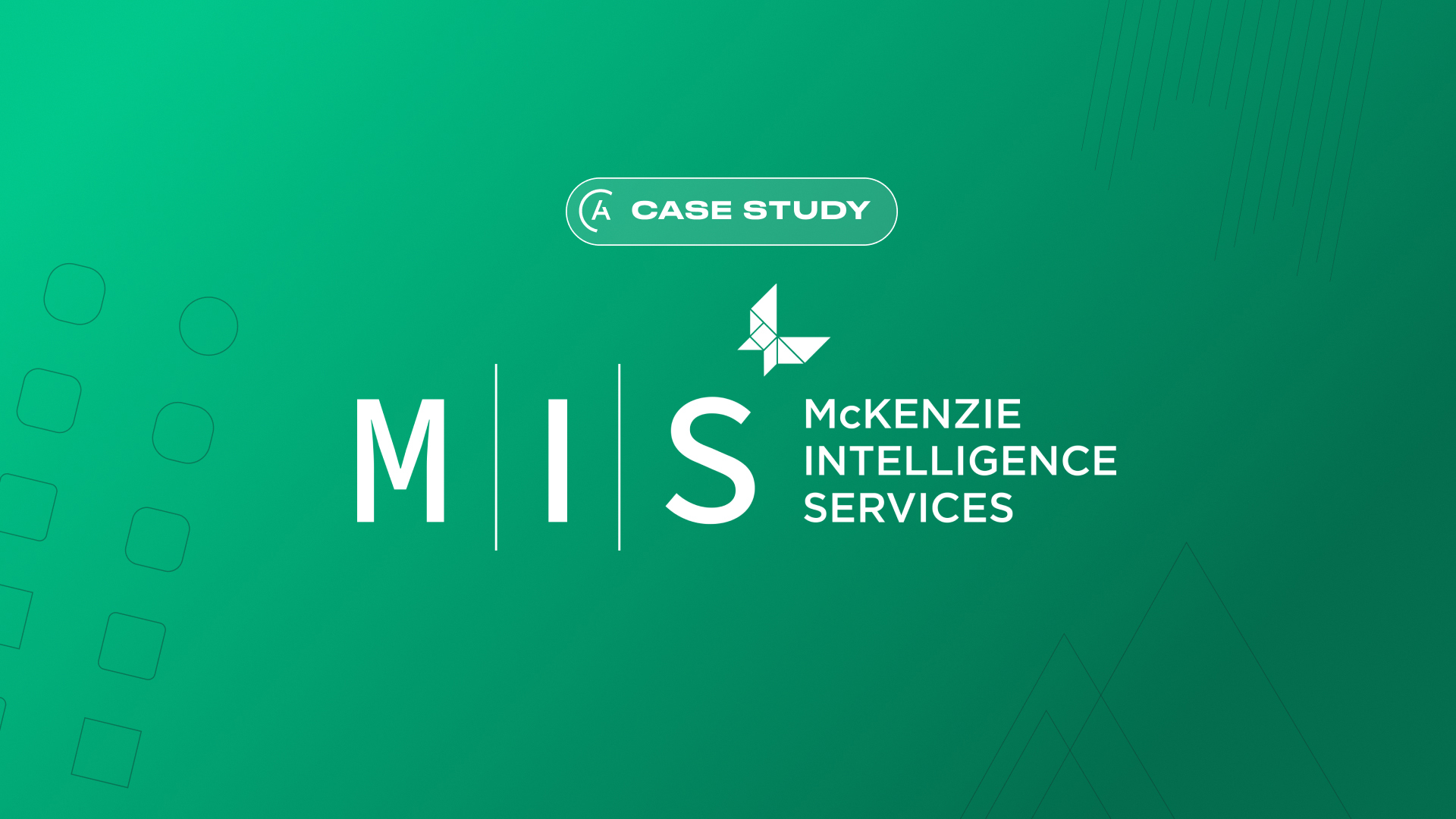
Introduction: The Challenge of Analyzing Disasters for Risk and Response
McKenzie Intelligence Services, a leading catastrophe assessment and intelligence company, specializes in providing risk assessment for natural and man-made disasters. The company analyzes global catastrophes, including hurricanes, floods, tornadoes, fires, protests, and wars. Andrei Nita, Head of Technology at McKenzie Intelligence Services, described their innovative approach as "Google Maps on overdrive," highlighting the company’s ability to overlay various data layers on a global map to provide comprehensive insights into ongoing events.
The primary services offered by McKenzie Intelligence Services include risk assessment, exposure assessment, and claims management. These services are critical for clients in industries such as insurance, government agencies, and NGOs, who rely on timely and accurate data to respond to disasters.
The company provides real-time, expert-validated assessments using satellite, aerial, and street-level imagery, combined with advanced analytics to determine the extent of damage to properties and assets. Data and workflow orchestration are vital to efficiently processing large volumes of imagery and data from multiple sources, ensuring accurate assessments.
The ability to automate and scale these processes is essential for handling the growing number of catastrophic events globally. Airflow powers various use cases, including ETL workflows for extracting data from multiple sources like NOAA and NHC, automated processing of large imagery files, machine learning pipelines for building damage assessment, and scheduling and automating previously manual tasks.
Astronomer has tripled our efficiency from a working hour perspective. There are no more working hours. Everything is just 24/7 now.
Andrei Nita
Head of Technology
McKenzie Intelligence Services
GEO - Revolutionizing Damage Assessment After Disasters
In addition to these services, McKenzie Intelligence Services has developed GEO, a groundbreaking platform that merges real-time data with expert analysis to create a single, reliable picture of an event's impact. GEO leverages cutting-edge technology like satellite imagery, social media, and sensor data to deliver comprehensive reports within a tight timeframe. Over 1,200 users across various organizations now rely on GEO, which has been used to assess over 150 global disasters, from wildfires to earthquakes. GEO offers industry-first features like detailed ground-level imagery, leading to faster and more effective post-disaster response across the globe. GEO is transforming how we understand and respond to crises, providing a vital tool for effective disaster management.
Initial Situation: The Manual Process and Its Pitfalls
Before adopting Astronomer, McKenzie Intelligence Services faced significant operational challenges due to their reliance on manual processes and ad-hoc scripts. These scripts were executed by individual developers, often on their personal machines, without a centralized system for tracking or managing workflows. This setup led to several critical issues. "Imagine uploading a large imagery file to GEO and then adding it to a map.Previously, this task had to be done manually, taking half a day or more."
Additionally, there was a lack of centralized tracking and logging. Without a unified platform to monitor and manage workflows, it was challenging to keep track of what tasks were running, their status, and their output. This absence of visibility made it difficult to identify and resolve issues promptly, leading to delays and inefficiencies. As Andrei mentioned,"I was unsure what was happening because there was nothing to see. I had to ask people what tasks they were running."
Data processing was also inconsistent and sporadic. With no standardized process in place, data was often processed on an ad-hoc basis, leading to variations in data quality and reliability. This inconsistency was problematic for clients who relied on accurate and timely data for critical decision-making. For example, hail data processing was done only when specifically requested by a customer, leading to gaps in data coverage.
The high operational overhead due to reliance on individual expertise was another significant bottleneck. Developers spent a considerable amount of their time managing and executing these manual processes instead of focusing on more strategic tasks. This inefficiency limited the company's ability to scale operations and respond to the increasing number of catastrophic events effectively.
These challenges collectively impacted McKenzie Intelligence Services ' business operations. Project timelines were frequently delayed due to the time-consuming nature of manual processes. Operational overhead increased as more resources were needed to manage these tasks. The company struggled to scale its operations and process high volumes of data consistently, which affected their ability to deliver reliable and timely assessments to clients. Additionally, the lack of data quality and governance impacted customer satisfaction, as clients depended on precise and current data for their risk assessment and claims management processes.
The volume of work output can be significantly higher, potentially 100 or even 1,000 times greater, since the only limitation is the resources allocated to running your workloads.
Andrei Nita
Head of Technology
McKenzie Intelligence Services
Decision Process: Leveraging Expertise with Astronomer
Facing the critical need to address their operational challenges, McKenzie Intelligence Services embarked on a search for a solution that could streamline and automate their data workflows. The goal was to find a platform that would reduce the reliance on manual processes, enhance data consistency, and improve overall efficiency.
Andrei, spearheaded the evaluation process, bringing his extensive experience with data engineering and workflow orchestration to the table. He had been an Airflow user for the past six years and had transitioned to using Astronomer’s managed Airflow platform four years ago. His deep familiarity with Airflow’s capabilities and Astronomer’s value proposition informed the decision-making criteria. Andrei noted, "I've been using Airflow extensively for a while now. When asked what other options there are on the market, I list the pros and cons of a few alternatives, and people often agree that Airflow is the best choice."
Having previously implemented Astronomer’s Airflow at other companies, Andrei was well aware of the benefits it could bring. He had seen firsthand how Astronomer simplified the deployment and management of Airflow, allowing teams to focus on developing workflows rather than maintaining infrastructure. This past experience was a significant factor in his decision to implement the same solution at McKenzie Intelligence Services.
During the evaluation process, Andrei considered several other options, including Prefect, which positions itself as an Airflow replacement. However, based on his extensive experience, he found that Prefect did not meet the operational robustness and ease-of-use criteria that were essential for his team. He briefly tested Prefect but quickly determined that Airflow was superior in handling their complex workflow needs.
Airflow, alongside Astronomer, emerged as the clear choice due to its comprehensive feature set, strong community support, and ease of integration with existing systems. The platform’s ability to automate and scale workflows effectively was a significant factor. Andrei was particularly impressed with the minimal infrastructure maintenance required and the responsive support provided by Astronomer, which assured him that any issues encountered would be swiftly addressed. He recalled, "The support is always responsive and knowledgeable."
The evaluation process included rigorous testing of Astronomer’s Airflow capabilities. Andrei and his team assessed the platform’s performance in handling their specific use cases, such as ETL workflows, large imagery file processing, and machine learning pipeline automation. The tests confirmed that Astronomer could meet and exceed the requirements of McKenzie Intelligence Services , offering a seamless transition from their manual processes to an automated, scalable solution.
Ultimately, the decision to transition to Astronomer’s Airflow-powered orchestration platform was driven by Andrei’s confidence in the platform, built on years of experience and successful implementations. He knew that this platform would address the critical pain points they had been experiencing and enable McKenzie Intelligence Services to enhance their operational efficiency and data consistency significantly.
A notable instance of this support was when a version stability issue arose. Time to remediation of critical infrastructure issues shrunk significantly to under 1 hour, managed by the Astronomer team.
Andrei Nita
Head of Technology
McKenzie Intelligence Services
Implementation: Transitioning to a Modern Workflow
The migration process from manual processes and ad-hoc Python scripts to an automated workflow orchestrated by Astronomer’s Airflow was a significant but well-managed transition for McKenzie Intelligence Services . With a clear understanding of the existing challenges and a defined goal to enhance operational efficiency, Andrei and his team embarked on this transformative journey.
The initial step involved a comprehensive assessment of the existing manual processes and scripts. Each workflow was analyzed to understand its dependencies, execution patterns, and potential areas for improvement. This thorough assessment was crucial for identifying the workflows that could be immediately transitioned to Airflow and those that required more complex reengineering.
With a detailed migration plan in place, the team began adapting existing scripts to run as Directed Acyclic Graphs (DAGs) within the Astronomer platform. This process involved breaking down the scripts into smaller, manageable tasks that could be orchestrated by Airflow. The team leveraged Astronomer’s extensive documentation and resources to ensure a smooth adaptation process. Andrei noted,"I asked my team to install the Astro CLI, check out the documentation, and get started. That was pretty much it!"
Throughout the migration, Andrei and his team benefited from Astronomer’s responsive support. A notable instance of this support was when a version stability issue arose. Time to remediation of critical infrastructure issues shrunk significantly to under 1 hour, managed by the Astronomer team. This level of support was a critical factor in maintaining momentum and confidence during the transition.
One of the significant challenges encountered was changing the team's mindset from a manual, ad-hoc approach to a structured, automated workflow. Effective documentation and training were pivotal in overcoming this hurdle. The team conducted regular training sessions to familiarize developers with the Astronomer platform and the new workflow processes. These sessions covered everything from basic usage of the Astro CLI to advanced DAG creation and management techniques.
The migration also involved setting up centralized tracking and logging within Airflow. This feature was a stark contrast to the previous lack of visibility and allowed the team to monitor the status of all workflows in real-time. By integrating Airflow’s logging capabilities with their existing monitoring tools, McKenzie Intelligence Services achieved a level of observability that was previously unattainable. Andrei explained, "Since Airflow was established, everything that happens is now triggered through Airflow. All of the logs appear in Airflow, if you want to consult what is happening or why a task is failing."
The ETL workflows were among the first to be automated, extracting data from multiple open sources like hurricane data from NOAA and the National Hurricane Center. Additionally, the team automated the processing of large imagery files. Andrei described, "Imagine uploading a large imagery file, maybe around one gigabyte, through a button on a website. This action triggers Airflow to start a pipeline, which might take 10 to 30 minutes. However, the website can't be blocked for that duration. Instead, Airflow handles the processing in the background and updates the database once the task is completed."
Machine learning pipelines also benefited from automation. Andrei explained, "We have a building damage assessment computer vision model trained on historical data, where our imagery experts have assessed the damage. This gives us an advantage over our competitors because our tagging was done by ex-military imagery experts, resulting in a hand-assessment accuracy of 95 percent or higher. Our model can achieve an accuracy of 80 percent or more."
Initially, the team ran four key DAGs that were critical to their operations. "The most important one handles the upload of data, which is essential for building reports on our GEO Platform," Andrei noted. "The other DAGs involve extracting raw data from the internet and processing building-level data, which can still be done manually if necessary." Moving forward, McKenzie Intelligence Services plans to implement more DAGs, particularly for processing customer data, which is currently done manually for 50 to 100 customers. This next project aims to orchestrate dynamic DAGs built from a list of different companies and their portfolios.
The implementation phase concluded with a thorough testing and validation process. Each migrated DAG was subjected to rigorous testing to ensure it functioned correctly and met performance expectations. This validation was essential for ensuring the reliability and accuracy of the automated workflows.
Overall, the transition to Astronomer’s Airflow-powered platform was a well-orchestrated effort that significantly improved McKenzie Intelligence Services ' workflow management. The combination of detailed planning, effective training, responsive support, and comprehensive testing ensured a successful implementation, setting the stage for enhanced operational efficiency and scalability.
Post-Implementation: Realizing the Benefits of Automation
The transition to Astronomer’s Airflow-powered platform has significantly transformed McKenzie Intelligence Services' operational efficiency and scalability. Andrei observed, "It has tripled our efficiency from a working hour perspective. There are no more working hours. Everything is just 24/7 now." This shift has ensured consistent data processing with significantly reduced human error, leading to better data quality and governance.
One of the most beneficial features has been the API-triggered DAG execution, which allows for automation of various tasks that previously required manual intervention. This capability has streamlined numerous processes, from data extraction to machine learning pipeline execution. The centralized logging and monitoring features of Airflow have provided the team with real-time visibility into all workflows, enabling prompt identification and resolution of issues.
Quantifying the improvements, Andrei highlighted a significant reduction in operational overhead. By automating previously manual tasks, the team has been able to focus on more strategic initiatives, resulting in enhanced productivity. The transition from ad-hoc insight generation to instant insight generation has also been a game-changer, allowing the company to provide timely and accurate assessments to clients.
Andrei highlighted that because of these limitations, "The volume of work output can be significantly higher, potentially 100 or even 1,000 times greater, since the only limitation is the resources allocated to running your workloads."
Additionally, “the automation of large imagery file processing that used to take half a day or more now runs in the background, freeing up schedules for more valuable tasks” Andrei explains.
The platform now handles an increased volume of data and workflows, with plans for further expansion. The team has adapted well, showing increased enthusiasm and productivity. The streamlined processes have allowed for more efficient task management and reduced manual workloads. The integration of Airflow has enabled the company to move from a reactive approach to a proactive and scalable operation, significantly enhancing their ability to respond to catastrophic events.
Long-Term Impact and Future Plans: Scaling for the Future
Looking ahead, McKenzie Intelligence Services plans to expand the use of GPU workers for faster processing of computer vision models and further automate manual processes to enhance data quality and governance. Andrei expressed interest in a feature Astronomer might release, which would allow for GPU workers, significantly reducing processing times for their computer vision models. He mentioned, "Currently, it takes about one hour to estimate damage for 40,000 buildings, but with GPU support, we could lower that by 10x or 20x."
The goal is to enable scalability on the damage assessment model side and critically review other manual processes within the business. This forward-thinking approach aims to further enhance the company’s capabilities in handling large-scale disaster assessments efficiently. Additionally, the company plans to expand its data catalog, regularly integrating new data sources such as fire, earthquake, and tsunami data to provide more comprehensive assessments.
One specific improvement is the automation of hail event data. Previously, the team would process hail events once or twice per year based on customer requests. Now, they run a daily task on Airflow that extracts all hail data, aggregates it, and defines severity levels. Andrei explained, "We have predefined severity levels for Hail. We capture all the data, aggregate it, and send alerts to our intelligence team. This enables us to create events proactively, rather than only when specifically requested by clients."
Reflecting on the transition, he noted the benefits of moving to an automated system sooner to avoid the inefficiencies and errors of manual processes. "The transition has been seamless, and the support from Astronomer has been exceptional, transforming our operations," he emphasized.