Top Data Management Trends for 2022
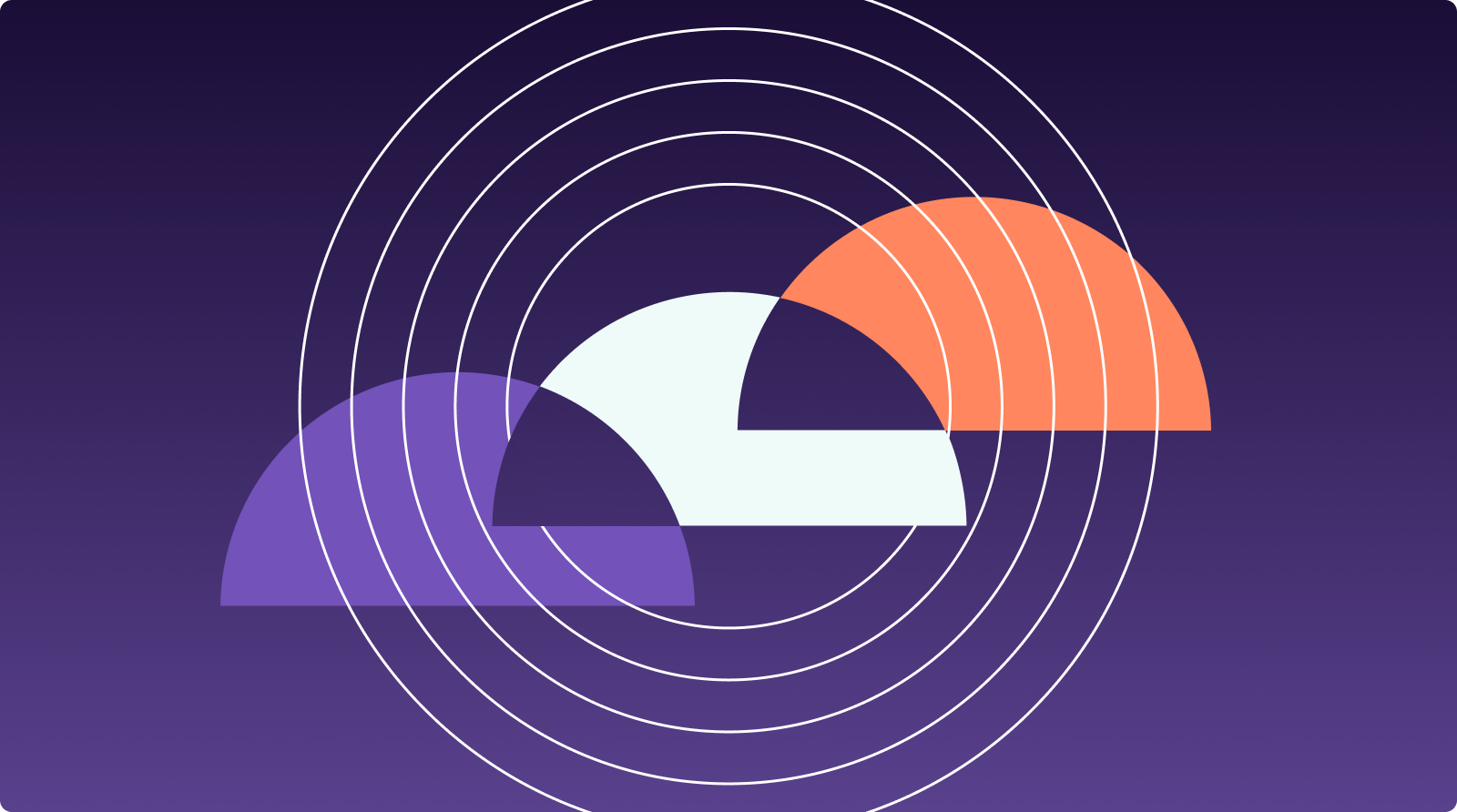
The adoption of cutting-edge systems, tools, and best practices can empower modern organizations, drive business, and allow for breakthroughs. It's no different in the data industry, where groundbreaking innovations emerge every few months.
A decade ago, distributed data management, which would enable large data workloads, was at the forefront of debate. By 2015 distributed systems were most commonly run using on-premises servers and clusters, as moving data to the cloud was just beginning to gain traction. Only last year, machine learning began entering a new era of simpler tools, requiring less sophistication to train and run. At the same time, advanced natural language processing tools like BERT and GPT-3 became more mainstream, generating exciting new approaches to augmenting language-oriented applications.
The world of data is ever-changing. At Astronomer, we see a mix of micro and macro trends on the horizon as we are not only close to the data management story, we co-write it by playing an active part in developing Apache Airflow® and shaping data orchestration space. In this article, we bring together nine experts from our team that offer an in-depth look into the most prominent trends and phenomena shaping the modern data world.
Key takeaways:
- Data lineage and data quality will be on everyone’s mind
- Data decentralization is here to stay
- Consolidation of data tools is coming
- Read on to learn more!
Data Meshes and the Human Element of Data
Roger Magoulas, Data Strategy in Engineering
Data lineage and data quality
The value of data improves the more you understand it and the more reliable it is. By correctly documenting and storing data, as well as ensuring reliability by moving towards reproducible pipelines and more formal analytics projects, you increase the productivity of your teams and eliminate data silos—all serving to deliver more focus on providing useful insights to the business.
Data meshes
Data meshes help eliminate silos between data teams, making sure that the experience and knowledge about data are shared among data professionals in the company. Data meshes are also about connecting platforms that those teams are using so data can be easily moved around for the benefit of the organization. Companies will try to find better ways of unifying and connecting the tools so that data professionals don’t have to context switch and work in a silo.
Data meshes provide a way to manage the tensions between decentralizing and centralizing data resources—where you decentralize somewhat, but you have a common infrastructure. At Astronomer, we believe data pipelines, when deployed to empower the entire data team, can be a significant accelerant to realizing a data mesh architecture.
Unified IDE tools for analysts
Data professionals often rely on a disconnected network of tools to get their work done, mixing notebooks with language-based IDEs, data management interfaces, data exploration tools, orchestration interfaces, and spreadsheets. We expect IDEs to evolve in a manner that provides a more common and consistent palette for getting analytic work done–helping reduce the costs of context switching between tools and increasing productivity.
Putting data in a human context
The 2000s were the time of building computation resources to handle big data. The 2010s were about creating techniques to make sense of data (such as machine learning or natural language processing). I think this decade is going to be more about making use of the data in a human context.
Looking only at numbers gets you nowhere. They only start to create a story when put in the perspective of qualitative data. That work requires more annotation—you have to think of a human element (that is less predictable than a machine behavior) to add a wider perspective and take full advantage of data. By adding the context, you’re making sure that your data is useful—to your business, users, and customers.
Productionizing and Decentralizing Data
Bolke de Bruin, VP of Enterprise Data Services
Enterprises today are moving to DevOps teams and product teams that are relatively decentralized. They are empowered to do end-to-end product development. It can be part of the product, but they retain end-to-end responsibility. They can iterate fast and create a tremendous amount of data too. A central data team cannot keep up with this. It’s moving too fast. Therefore, the responsibility of maintaining datasets is shifting from the central teams to the product teams.
It’s also worth to mention the productionization of data. I think that building operational, scalable, observable, and resilient data systems is only possible if the data itself is treated with the diligence of an evolving, iterative product. This requires tooling that is inherently data-aware. Data discovery with tools like Datakin and Amundsen can make transparent what data is driving your revenue. Integration of data quality tools like PopMon and Great Expectations helps you monitor data and stay within compliance boundaries. Having all these things in place can greatly speed up your business processes.
Learn more about the future of data in the interview with Bolke.
Specialization of Tools Through a Partnership with Domain Experts
Santona Tuli, Staff Data Scientist
Today’s no/low-code solutions promise to abstract away most of the dynamics of data and ML pipelines from data professionals, but this creates a glaring lack of domain expertise. We’ll see a shift towards more involving but also more specialized and performant tools in the data space. These will allow the domain expertise that data professionals bring to enrich data as a product and the products that data support. In other words, decentralized, versatile, and empowered teams rather than apparently comprehensive tools will help unlock value from data.
Particularly in the emerging field of data quality, tools will evolve and support the extensive exploration and forensic study of data that data professionals perform, not attempt to replace them with automated ‘holistic’ data quality solutions which are necessarily sub-par due to the oxymoronic nature of the charter. Today it is easy to automate basic data quality checks—such as ensuring the consistency of the data type within a column—but checks on the aggregate data and the underlying distributions require a human with deep domain knowledge and statistical understanding.
In the near future, specialized tools will coalesce into comprehensive ecosystems where data will flourish as a first-class entity, stewarded by empowered data professionals working together.
The Proliferation of Tools and Services in the Space
Jarek Potiuk, Apache Airflow® PMC Member and Committer, Technical Advisor at Astronomer
We will see a greater proliferation of data-related tools. For example, more specialized databases designed for a specific use case. We already have a time series database (for processing data that changes over time) or a graph database (for storing information about relationships between data points). While they haven't received much attention in the past, this could change in 2022.
This will introduce some new challenges, as most businesses have more than one use case, which means data teams will need more than one database (or at least more than one way to interact with data). They will have to efficiently connect their databases, combine the data, present it in a unified way, and draw accurate conclusions.
Databases are just one example of this. There are so many new tools, products, and services on the market that data professionals will need to be able to connect in a unified manner, even if they are from different ecosystems. The good news is that Apache Airflow®, as a fully customizable orchestrator, can act as the glue that allows systems to communicate smoothly.
Data Management: MLOps, DataOps, and Data Pipelines as a Network of Interconnected Processes
Steven Hillion, VP of Data and ML
Organizations are viewing data orchestration with increased urgency, realizing that it’s a critical part of their operational infrastructure. They need to manage data, understand the relationships between data, fix problems, and deliver operational analytics to the front lines of the business.
Instead of scheduling data pipelines with cron jobs, Control-M, and one-off solutions, companies today need to view orchestration as a fundamental component of running the business and focus on the concept of data pipelines as a network of interconnected processes (as opposed to data pipelines managed by disparate technologies and separate teams).
Additionally, more companies integrate data management with MLOps. We can see DataOps and MLOps teams coming together to create pipelines that extend all the way from raw ingestion, through the feature generation, to the model training and model monitoring. It’s a trend towards a comprehensive ecosystem of data pipelines and models. At Astronomer, we see it first hand—our most sophisticated clients are the ones who treat Airflow as part of a single ecosystem of data management — all the way from data to models to action.
Easier Adoption of Data Management Tools
Kenten Danas, Field Engineer
I think in 2022, there will be an increased focus on making the adoption of data management tools easier. The data ecosystem is massive and will continue to grow as more specialized tools are developed for certain use cases, and it’s an unreasonable ask for data engineers to be deeply proficient in all of them.
One of the major concerns we hear about from the Airflow community is that when somebody leaves the team they lose the critical knowledge to running their data pipelines. I believe we’ll see a lot of work towards lowering the barrier to entry with commonly used tools, as well as an effort to make different tools play together more seamlessly so that anybody with a background in data engineering can figure out how to tie together the right tech stack for their team.
One trend specifically related to Airflow, that might continue next year, is the expansion of the provider network — we’ll have better integrations with common tools that may be possible with some workarounds today but are not yet in an ideal state.
Data Lineage and Data Quality
Pete DeJoy, Founding Team, Product
One thing that’s certainly worth mentioning is all of the activity around data lineage. The Cambrian explosion of tools comprising the “modern data stack” and the push for embedded, decentralized data resources across an organization have made it more critical than ever to have a cohesive, end-to-end view of data asset lifecycles.
If you have a reporting view in Snowflake that is updated daily by ten separate processes (Airflow, dbt, BI queries, etc) and one of those jobs fails, downstream dashboards and reports will be built on top of “bad data.” Viewing the world through an asset-first lens is a natural next step to viewing the world through a process-first one; if you have a single pane of glass for all of your data assets and the processes running against them, you can quickly and easily track down upstream failures that are adversely affecting your data quality. At the end of the day, we all want to rest assured that our executive KPI reports and dashboards haven’t been silently corrupted due to an intractable failure somewhere in the supply chain. The folks behind OpenLineage are doing some really great work here to build a standard spec and framework for data lineage collection that integrates with a variety of cataloging and lineage systems.
Another point of interest is the buzz around data quality. Airflow and other process-driven orchestration systems are very good at monitoring your process state, but you may want to drill deeper into whether the data you’re ingesting fits within a certain confidence interval. This kind of exposure allows you to build systems around validation and quality checking, so you can rest assured that the dashboard you’re presenting to your CEO isn’t built off of corrupt data. Great Expectations is a tool to take a look at in this space. We also support first-class integration with their libraryvia our provider if you’re interested in baking quality checks into your Airflow DAGs.
Importance of Integrations & Standards Between Tools
Paola Peraza Calderon, Founding Team, Product
If we've learned anything from the evolution of software and data over the past 10 years, it's that we'll be on this data journey for far longer than it took us to get to the obvious ubiquity of software engineering as a discipline. Take a look at this conversation between Martin Casado from a16z and Tristan Handy at Coalesce from DBT Labs. The general need to extract value from data is arguably more akin to philosophy than it is engineering – so complex, layered, and human that we'll be ideating, building, and rebuilding systems for a long time. This is exciting, and it means that we're all building careers with some serious long-term gains in sight.
Given that premise, I think the pressure for our technology to break down barriers will only continue to grow in 2022. This can mean a few things:
- Data orchestration will continue to be at the center of the modern data stack. Without a scheduler, your system falls apart. Any mention of data quality, data governance, and data lineage is impossible without data orchestration.
- Tighter integration between tools. Data practitioners will expect that using Apache Airflow® with DBT, Datakin, Snowflake, Datadog, and any sort of database is actually easy. At Astronomer, we have dozens of daily conversations that go something like, “How do I use Astronomer and [insert-most-things-here]?” or “I tried to install x version of this library but it doesn’t work with [insert-a-lot-of-things-here].” Product leaders - data tools don’t work in isolation. Compatibility, documentation, and accessibility will be key.
- The continued importance of strong, equitable developer communities in Slack, in-person conferences, and otherwise that give space to dissonance and productive disagreement while keeping us (and our job descriptions) moving in the same general direction.
This all doesn't mean that one tool will "rule them all" or that role specializations will go away – it just means that we'll be building and using sophisticated technologies that have to talk to each other and speak the same language. Open source and open standards make this promise that much more compelling and feasible.
Data Visibility and Governance
Maggie Stark, Data Engineer
There’s going to be more focus on data visibility and governance. Seeing the history of your data but also your analytical models (machine learning models can often be obscure and hard to figure out) will become essential. Especially now, when there are regulations put in place to track and use data correctly if you can't tell what a computer is doing with your data those regulations are going to be so much harder to follow.
The industry has done a lot of work with optimizing data—how to store it and how to analyze it. Now it’s time to focus on scaling data quality, visibility, and interpretability practices. Ultimately, better understanding data by knowing the lineage of how, when, and why your data changed, creates a richer and more complete picture that allows for better, more informed decisions.
Get in touch with our experts to discuss further how orchestration can accelerate your data strategy in 2022.