3 Key Takeaways from Airflow Summit 2023
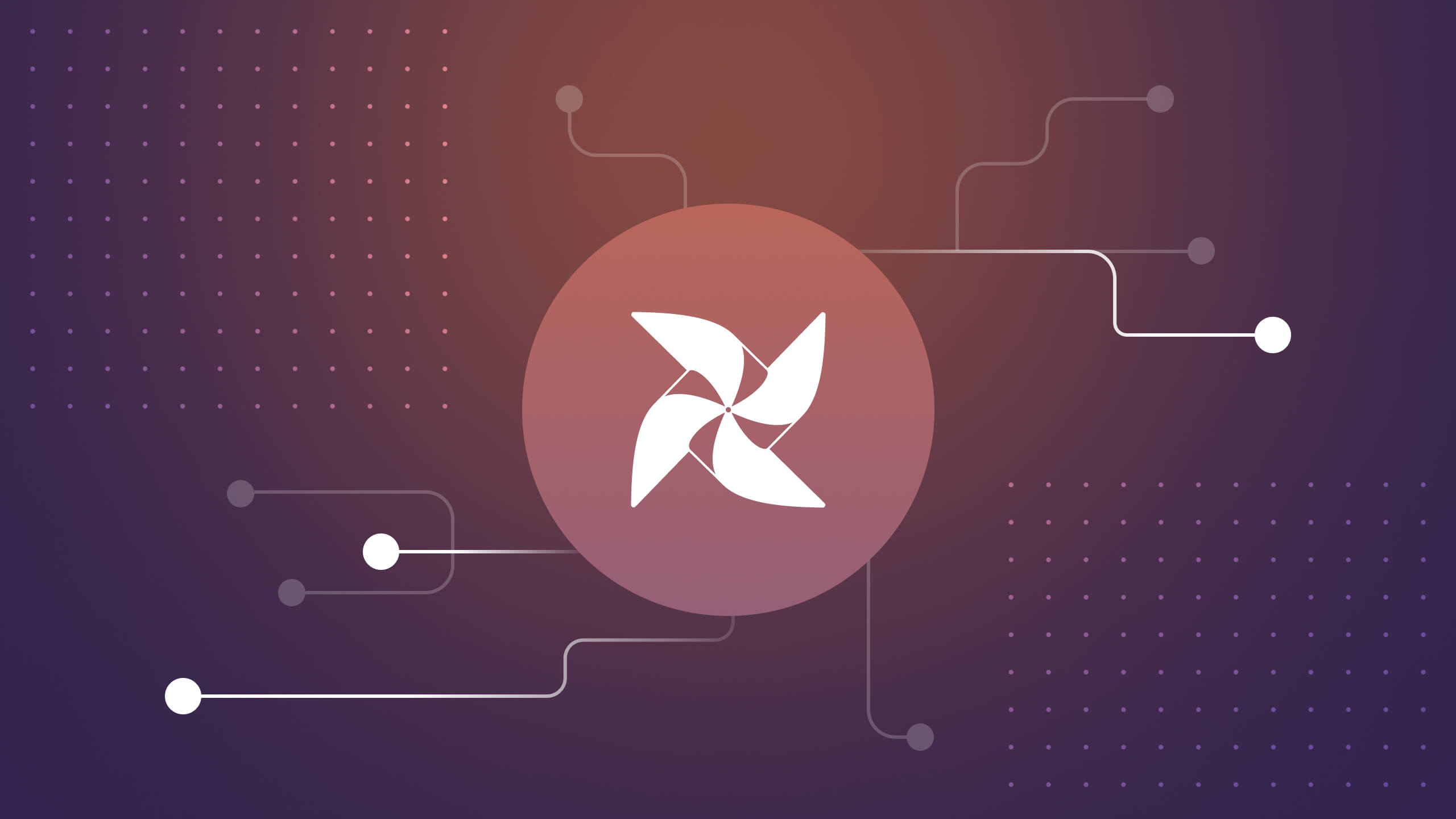
Last week, the first-ever in-person Airflow Summit occurred in Toronto, Canada. Over 500 attendees from 20+ countries came together for all things Airflow, orchestration, and open source.
It’s tough to describe the energy and the vibe of the conference to someone that wasn’t there. Between all the hallway conversations, talks, impromptu meetups, and workshops, a few things really stood out:
1. Airflow is the centerpiece for all types of data architectures.
Regardless of the specific tooling they are using, data teams will often implement higher level patterns around things like a data mesh, the notion of “data contracts,” or a way to operationalize data quality with Airflow. Whatever the right pattern is for a data team, Airflow Summit showed that DAGs are usually at the center of it. We heard from folks like Delivery Hero and Kiwi.com on the data mesh in particular, and different ways Airflow can be used to power decentralized teams to all move quickly. PepsiCo and Acryl Data spoke to the business criticality of accurate data, and how they’re able to serve that to the business with data contracts and data quality frameworks. And when data is moving around, lineage becomes crucial – folks from Google, Astronomer, and others all spoke to the benefits of OpenLineage. These talks really highlight that:
- Data teams connect the different parts of a business together
- There’s no “one size fits all” solution to how a data team should operate
- Airflow really is the “central nervous system” for a business
2. Airflow is definitely a machine learning orchestrator.
It’s not clear if there will ever be a consistent definition of “MLOps,” what the difference between feature engineering and data processing is, or how much of machine learning engineering is really just data engineering. What is clear is that regardless of definitions, Airflow is at the center of the AI/ML conversation. Sessions from places like The Home Depot, and Faire showed that adopting Airflow for base data engineering workloads allows for a foundation to build upon for doing all sorts of AI-centric initiatives around machine learning, and other forms of predictive analytics. The explosion of Large Language Models (LLMs) and foundational models is accelerating this – and it’s clear why! Astronomer’s Julian LaNeve and Kaxil Naik showcased exactly why Airflow was mentioned in a16z’s Emerging Architectures for LLM Applications with Ask Astro, a reference implementation that shows how Airflow can be used to power data Retrieval Augmented Generation (RAG) apps.
3. Open source is awesome.
Far and away, the best part about Airflow Summit was the community. Open source is a wonderful thing, bringing together folks from all around the world and creating a sense of shared ownership and belonging. Airflow Summit didn’t just bring together the Airflow community, it brought together folks from the communities behind OpenLineage, dbt, Metaflow, Datahub, the major clouds, and so much more. The discussions on what's changed over the years, where the project has been, and how the community can better serve the users set the stage for the next set of great users and stories.
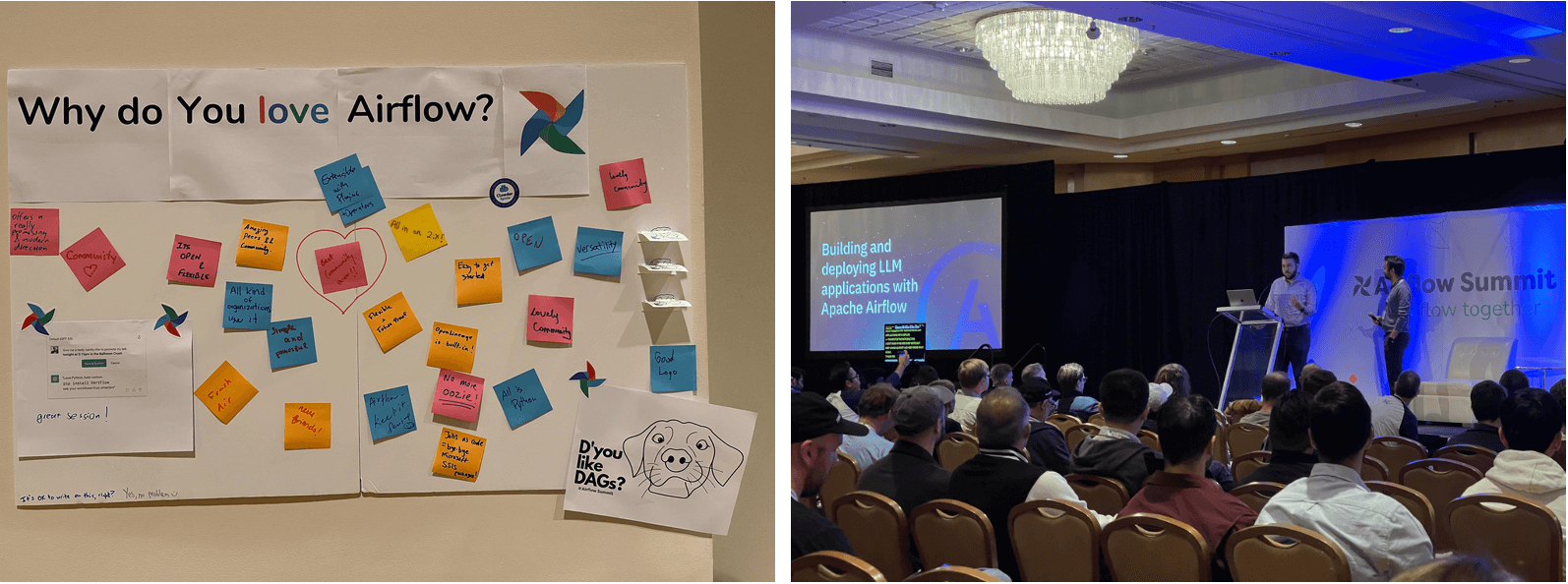
The global Airflow community coming together in person for the first time was truly special. If you were not able to make the trip to Toronto, or if there were sessions that you had to miss, you’re in luck as all the talks from Airflow Summit will be released on YouTube over the next few weeks.
📣 Let’s chat Airflow 📣
Want to learn more about Airflow? Reach out to us directly! We're here to engage, discuss, and explore more with you.